Case Studies
- Home
- Case Studies
Fraud in Auto Finance Case Study
This case study is related with auto finance fraud. Here we managed to cut down the losses of due to repossession and fraud by $3mm on 50% test population within 6 months after machine learning model implementation. The model was built to identify the fraud customer early and do early repossession in such cases while give more time to pay to highly probable honest customers.
The key model variables were as follows:
Prime / Non-Prime Customers
Times 90+ in Last 12 Months
Internal Behaviour Score (Observation Month)
Equity at Risk
Days Since Last Contact
Fraud or Loss in Credit Cards Case Study
This case study is related with fraud is credit cards. While onboarding Customers, Bank Identified Customers as Prime (with good credit standing) and Sub-Prime (with poor Credit History or Bureau Score), these Sub-Prime Customers are mostly rejected by other lenders and this is sizeable market and opportunity for the Bank to offer Credit Card (with lower Credit Limit and harsher punishment for default) and as such it is expected that Default/Losses and Frauds would be higher from this Segment. Prior to Mid Stage Risk Based Model Development the existing model was based on Origination Characteristics (When Customer Applied for Credit Card) and Bank continued to maintain separate Collections/Recovery Agencies (both Internal and Off-Shore Outsourced) for Prime and Sub-Prime Customers respectively.
New Risk Based Model aims do away with this barrier# and optimise recovery efforts, Thus High-Risk Accounts (Prime or Sub-Prime) would be treated with High Intensity throughout delinquency window. Model resulted in $7 mm+ Cost Savings in 6 months of Model Implementation
Fraud in Auto Insurance Case Study
Due to ineffective Fraud Detection and Prevention Techniques, the average TAT for claim processing is significantly on the rise. Further to make matters worse, there is steady increase in claims filing, and this claim set contains a significant Fraud Subset. Thus, the necessity of investing in effective and efficient model building and continuously improving to ensure relevance.
New Model developed with the below objectives:
a) To Identify fake or superficial road accident Insurance claims and Reject such customers.
b) Extensive focus on variety of risk trajectories to identify Patterns and append to existing rules.
Model helped to reduce the Fraudulent claim’s approval reduced by ~50% within 6 months of Strategy Implementation.
Fraud in Health Insurance Case Study
This case study is related with fraud is health insurance claims. A Claims Constraint Scoring Mechanism is required to be implemented which would Identify (with a significant level of accuracy) the Fraudulent Claims from the Genuine Claims. This Scoring methodology developed from historical Claims information would be effective in Rejecting Fraudulent Claims. A model was built using historical information. Following variable were found significant:
- Reimbursement Amount
- Count of Number of Third Parties
- Number of Reimbursed Claims
- Detection Trend
- Procedure code
- High Cost Claims
Result was with 20% Test Population, ~ $600K worth of Fraud Claims Rejection in 3 months of model implementation.
Fraud in Home Insurance Case Study
This case study is related with fraud is home insurance claims. Homeowners carry insurance on their property so they can submit a claim if damages result from a covered risk. It’s reassuring to know that if your house burns down or suffers damage by an ‘Act of God’, you won’t have to start over again.
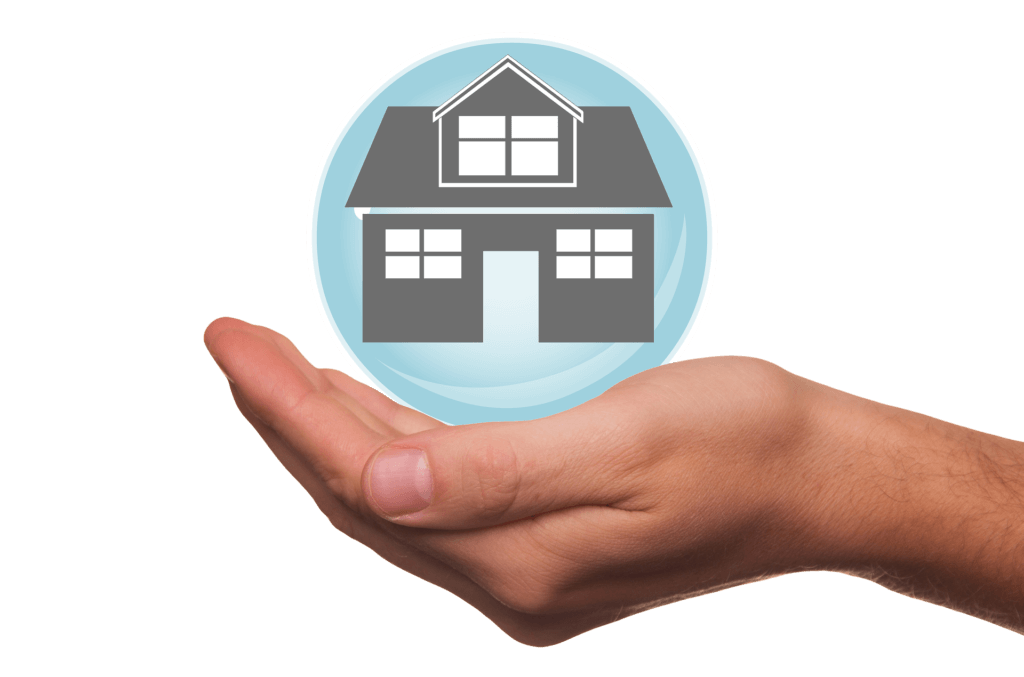
Rule Engine Based Implementation For Fraud Management
We have inhouse expertise available for implementation of expert based rule management system for fraud management. We can help with rule management implementation for IBM’s ODM and FICO’s Blaze advisor for fraud management.
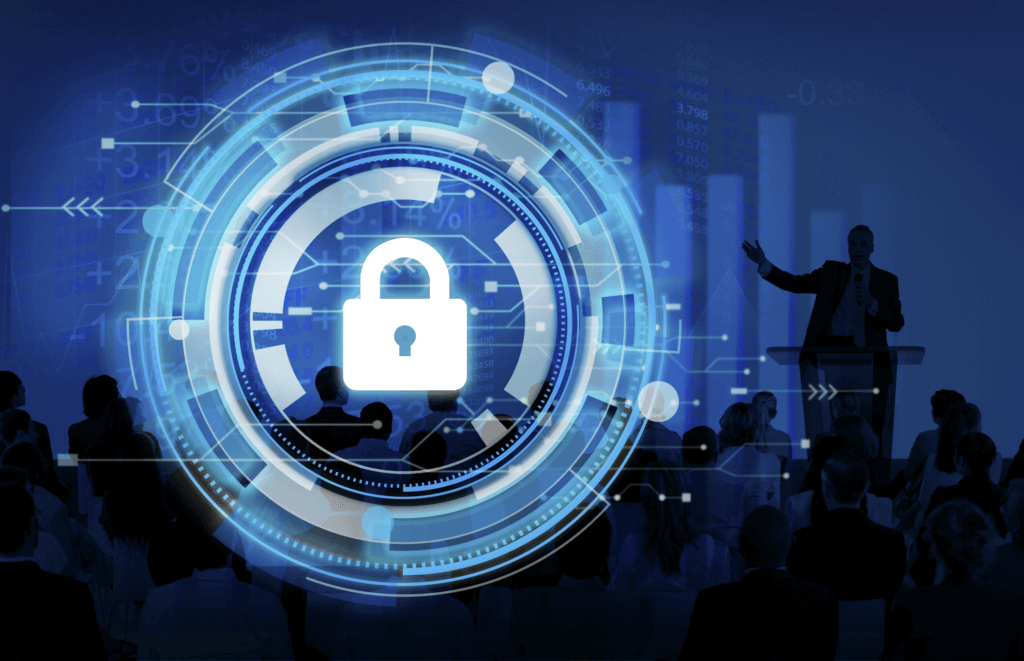
Benefits:
Benefits:
- FRAUD IN AUTO FINANCE : MODEL DECLARED CHAMPION WITH $3 MM+ LOSS REDUCTION IN 6 MONTHS OF STRATEGY IMPLEMENTATION
- FRAUD OR LOSS IN CREDIT CARDS : MODEL DECLARED CHAMPION WITH $7 MM+ COST SAVINGS IN 6 MONTHS OF IMPLEMENTATION FROM 40% TEST POPULATION
- FRAUD IN AUTO INSURANCE : FRAUDULENT CLAIMS REDUCED BY ~50% WITHIN 6 MONTHS OF STRATEGY IMPLEMENTATION
- FRAUD IN HEALTH INSURANCE : WITH 20% TEST POPULATION, ~ $600K WORTH OF FRAUDULENT CLAIMS REJECTED IN 3 MONTHS OF RULES IMPLEMENTATION.
- FRAUD IN HOMEOWNERS INSURANCE : WITH REDUCTION IN INSURANCE PREMIUM, INCREASED INSURANCE SALES